In recent years, Bayesian methods have gained popularity in the field of housing investment forecasting. These methods, which are based on Bayes’ theorem, allow for the incorporation of prior knowledge and uncertain parameters in the forecasting process. Two specific methods that have been widely used in this context are Bayesian Vector Autoregression (BVAR) and Factor-augmented Vector Autoregression (FAVAR). In this blog post, we will delve into the details of these two methods and discuss their advantages and limitations in the context of housing investment forecasting.
Bayesian Vector Autoregression (BVAR)
BVAR is a Bayesian version of the popular Vector Autoregression (VAR) model. The VAR model is a multivariate time series model that is used to describe the dynamic relationships among multiple variables. In the context of housing investment forecasting, a BVAR model may be used to examine the relationship between housing prices and various economic and demographic factors.
The key advantage of BVAR is its ability to incorporate prior knowledge about the parameters of the model. This is done by specifying prior distributions for the parameters, which can be updated as new data becomes available. This allows for more robust and accurate forecasting, as it takes into account the uncertainty surrounding the parameters.
However, one limitation of BVAR is that it assumes that the variables in the model are stationary. This means that the mean and variance of the variables do not change over time. If this assumption is violated, the model may produce inaccurate forecasts.
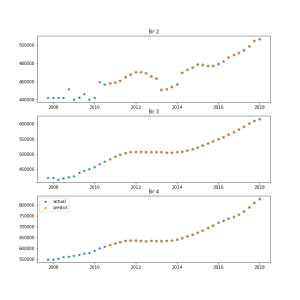
Factor-augmented Vector Autoregression (FAVAR)
FAVAR is an extension of the BVAR model that incorporates a small number of latent factors. These factors are unobserved variables that are thought to drive the dynamics of the observed variables. In the context of housing investment forecasting, these factors may include economic conditions such as GDP growth and interest rates.
The key advantage of FAVAR is that it allows for a more parsimonious representation of the underlying dynamics of the data. By incorporating latent factors, it reduces the number of parameters that need to be estimated, which can improve the model’s forecasting performance.
However, one limitation of FAVAR is that it may be difficult to identify the latent factors. This is especially true in the case of housing investment forecasting, where there may be a large number of potential factors that could drive the dynamics of housing prices.
Conclusion
Bayesian methods, such as BVAR and FAVAR, have proven to be powerful tools in the field of housing investment forecasting. They allow for the incorporation of prior knowledge and uncertain parameters in the forecasting process, which can lead to more robust and accurate forecasts. However, there are also limitations to these methods, such as the assumption of stationarity in BVAR and the difficulty of identifying latent factors in FAVAR. Despite these limitations, the use of Bayesian methods in housing investment forecasting is a promising area of research that is worth exploring further.